Made by Lullymore-west
“This should only take 30 minutes”
As part of the 13-week “Data Engineering in Python” bootcamp with Northcoders, we worked on developing an Extract, Transform, Load (ETL) pipeline for the fictional company Totesys. The goal was to automate data ingestion, transformation, and loading using AWS services and infrastructure as code. Our pipeline was designed to run on a 30-minute schedule, with each step orchestrated by EventBridge. The plan involved extracting data from an RDS operational database using a Lambda function, with credentials securely managed in Secrets Manager. The extracted data would then be stored in S3 as JSON before undergoing transformation via another Lambda function, which used Pandas to parse and convert the data into Parquet format within a specified star schema. Parameter Store was used to manage parameters for these processes. Once transformed, the data would be stored back in S3, and a final Lambda function would load it into a remodelled Data Warehouse for future business intelligence analysis. CloudWatch was also set up for monitoring and logging. However, due to time constraints, the full implementation of the pipeline was not completed.
The Team
Iris Araneta
Iris is a licensed pharmacist transitioning into data,…
driven by a passion for technology and its impact. Iris’s interest grew while working with AI engineers, sparking an interest in Python-driven technologies and data driven healthcare solutions. To support this shift, Iris completed the Northcoders data engineering bootcamp, gaining hands-on experience and eager to apply these skills to develop impactful data solutions and drive business insights.
Ollie Lawn
With a degree in physics and astrophysics, Ollie has…
transitioned into data engineering, with a long-term goal of moving into data science and working in machine learning and AI. A dedicated musician with a passion for music production, Ollie also has a deep love and respect for the arts, with particular interests in photography and videography.
Callum Bain
A Junior Data Engineer who recently completed the Data…
Engineering bootcamp at Northcoders. With nine years of experience as a Telecoms Field Engineer, he developed a passion for data, recognizing its value in improving task success. Excited to leverage an engineering background and technical skills, Callum is eager to contribute to the field of Data Engineering.
Dani Ghinchevici
Dani is a newly qualified Data Engineer with a solid…
foundation in Biopharma. She is passionate about Big Data, ML, Digital Twin Tech, and Clean Tech. Her analytical mindset makes her adept at problem-solving and solution implementation. With a dedication to continuous learning and a keen eye for detail, she is well-equipped to drive impactful data-driven solutions in her field.
Lucy Milligan
Lucy is a career changer to the world of Software/Data…
Engineering, having worked in a previous role as an Engineering Geologist for over 5 years. Within this role, the projects she enjoyed most were those with large sets of data that she could get stuck into. Lucy subsequently started learning python and enjoyed the problem solving and logical nature of coding, leading her to complete the Northcoders bootcamp. She has a keen eye for detail and is never afraid to ask questions.
Joss Sessions
Previously a video designer for live events including…
music, theatre, opera and dance, Joss has spent the last seven years running a micro company exploring how to contextualise data flows to the point of interaction while caring for close family relatives as an unpaid carer. Joss’ intention post course is to combine his theoretical cyborg anthropology research with industry standard data practices to address some of societies biggest challenges.
Tech Stack
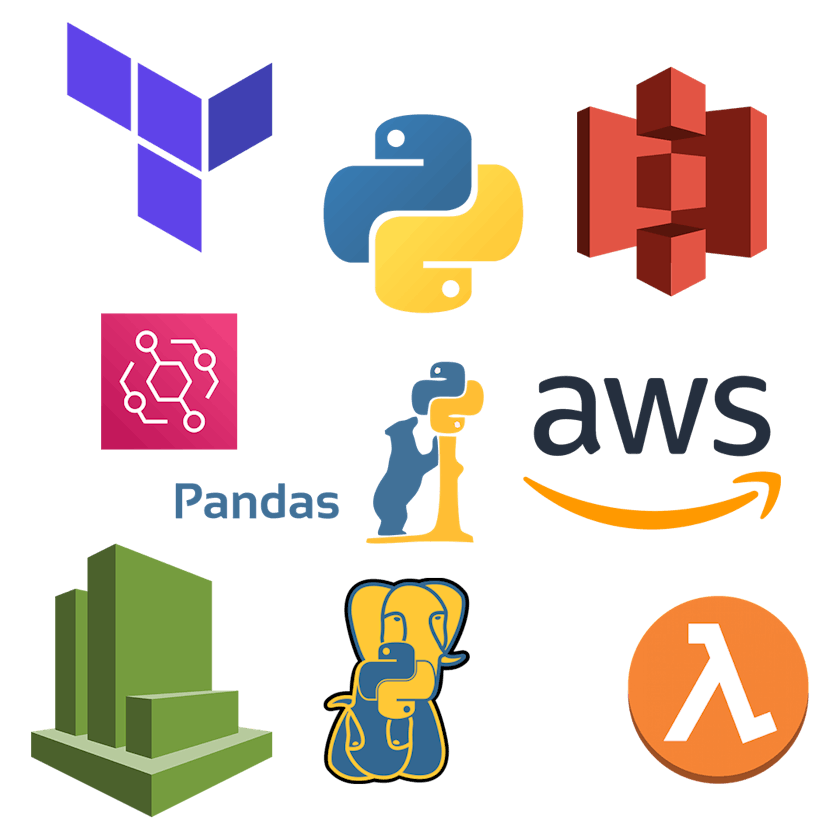
We used: Terraform, AWS (S3, Eventbridge, Cloudwatch, Lambdas, Secrets Manager, SSM Parameter Store), Python (including Pandas, Psycopg2, FastParquet), GitHub Actions Terraform: Used for infrastructure-as-code (IaC) to ensure reproducibility and automated deployment. S3: scalable data storage. EventBridge: to automate the workflow and trigger lambda functions from s3 “PutObject” notifications. Although we initially tried using a State Machine, we transitioned to using EventBridge for ease of deployment. CloudWatch: logging and monitoring of the lambda functions behaviour and overall pipeline execution. Lambda: Allowed us to write python applications that could be triggered by events, with associated utility functions for the ingest, transform and load stages of the pipeline. It’s also serverless and therefore cost efficient. Secrets Manager: helped manage credentials securely SSM Parameter Store: helped us keep track of the behaviour within the lambda functions (e.g. storing of timestamp variables needed to check if the database has been updated since the last invocation). Python: core programming language for data extraction, transformation, and loading (ETL). Pandas: used for data manipulation and transformation, using the ingested data in JSON format. Psycopg2: used for interacting with the PostgreSQL Totesys database, hosted in AWS. This was chosen for its efficiency and versatility. Fast Parquet: used for efficient storage in Parquet format and is a smaller package size than Pyarrow (smaller size required for the Lambda layers). GitHub Actions: continuous integration and deployment (CI/CD). Ensured automated testing, security checks and PEP8 compliance. This was also used to deploy the terraform code.
Challenges Faced
Given it was the groups first time creating an ETL pipeline, there were numerous challenges we faced, including: AWS Terraform configuration: Small oversights in our Terraform scripts led to unexpected difficulties. Transitioning from using a State Machine to EventBridge trigger Lambda response formatting: troubleshooting the response formatting for unpacking of data by second lambda Pandas layer attachment: Identifying and attaching a suitable AWS Lambda layer that hosts the Pandas library proved to be a challenge. Parameter Store and Secrets Manager: Managing sensitive information securely using AWS Parameter Store and Secrets Manager was essential but complex. Testing: testing the interaction of the lambda functions with operational databases (unit testing vs integration testing) Completing the project within 2 weeks We are really pleased with how we worked together as a group and the progress we made, despite not completely finishing the project. Although we have learnt a lot over the course of the bootcamp, the project also highlighted how much more there is to learn within the data engineering field!