Made by Study BuddAI
An on the go learner App
Our app makes studying more effective by transforming your study notes into custom multiple-choice quizzes using Gemini AI. It takes your uploaded PDF notes, processes them, and generates quizzes that challenge your understanding of the material. By actively testing your knowledge, the app enhances your study sessions and helps you retain information more effectively. The idea for the app stemmed from the need for a more interactive study tool. Traditional study methods like reading through notes or watching videos are often passive. We wanted to create a way to turn those materials into active learning experiences. Using AI, we built a tool that can automatically generate quizzes based on your study notes, making studying more engaging and personalised. User Experience: First, the user either logs in or creates an account. After logging in, the user clicks on Generate Quiz to begin the process. The user uploads their study notes in PDF format. The Gemini AI generates a multiple-choice quiz based on the content. After the quiz is created, the user goes to the quizzes section and can begin answering the questions. Once finished, the user can see how many questions they answered correctly and which ones they got wrong and retake the quiz if you need more practice on certain areas. This process helps you actively learn, track your progress, and focus on areas where you need improvement. With the combination of AI-powered quiz generation and immediate feedback, the app makes your study sessions more efficient and engaging.
The Team
Emer Rafter
Senior QA Engineer turned Software Developer with 5 years…
of experience in testing and a recent full-stack JavaScript bootcamp at Northcoders. Skilled in React, Node.js, Cypress, and test-driven development, now focused on leveraging my QA background to deliver high-quality software solutions.
Joshna Joshy
JavaScript Developer career switcher. Seeking opportunities…
as a Junior Software Developer.
Nina Thomas
With a background in healthcare, I am making the transition…
into the tech space. I have a passion for creating simple, effective solutions to complex problems. I bring a range of crossover skills from my past experience, enhancing my approach to problem-solving and teamwork. I’m looking for a junior developer role where I can bring my creative thinking and team spirit to the table. I really enjoy environments that encourage growth and learning. With my varied background, I’m ready to contribute while continuing to develop my skills.
Nimasha Karanahalage
Software Developer with experience in Go, JavaScript,…
TypeScript, React, and databases like MySQL, PostgreSQL, and MongoDB. Skilled in building efficient, reliable applications and optimising processes. Experienced with Docker, Kubernetes, CI/CD, and cloud platforms (Azure, AWS). Focused on writing clean code and continuously learning new technologies.
Jay
Ahoy-hoy I’m Jay, a beginner developer. Before the bootcamp…
I tried my hand at learning C# once upon a time. Living in code purgatory I decided I needed to be pushed and tested, to be in a learning environment. So once I heard about Northcoders I applied to their Bootcamp. Experience here has been great. Been exposed to new technologies and approaches I never knew about.
Tech Stack
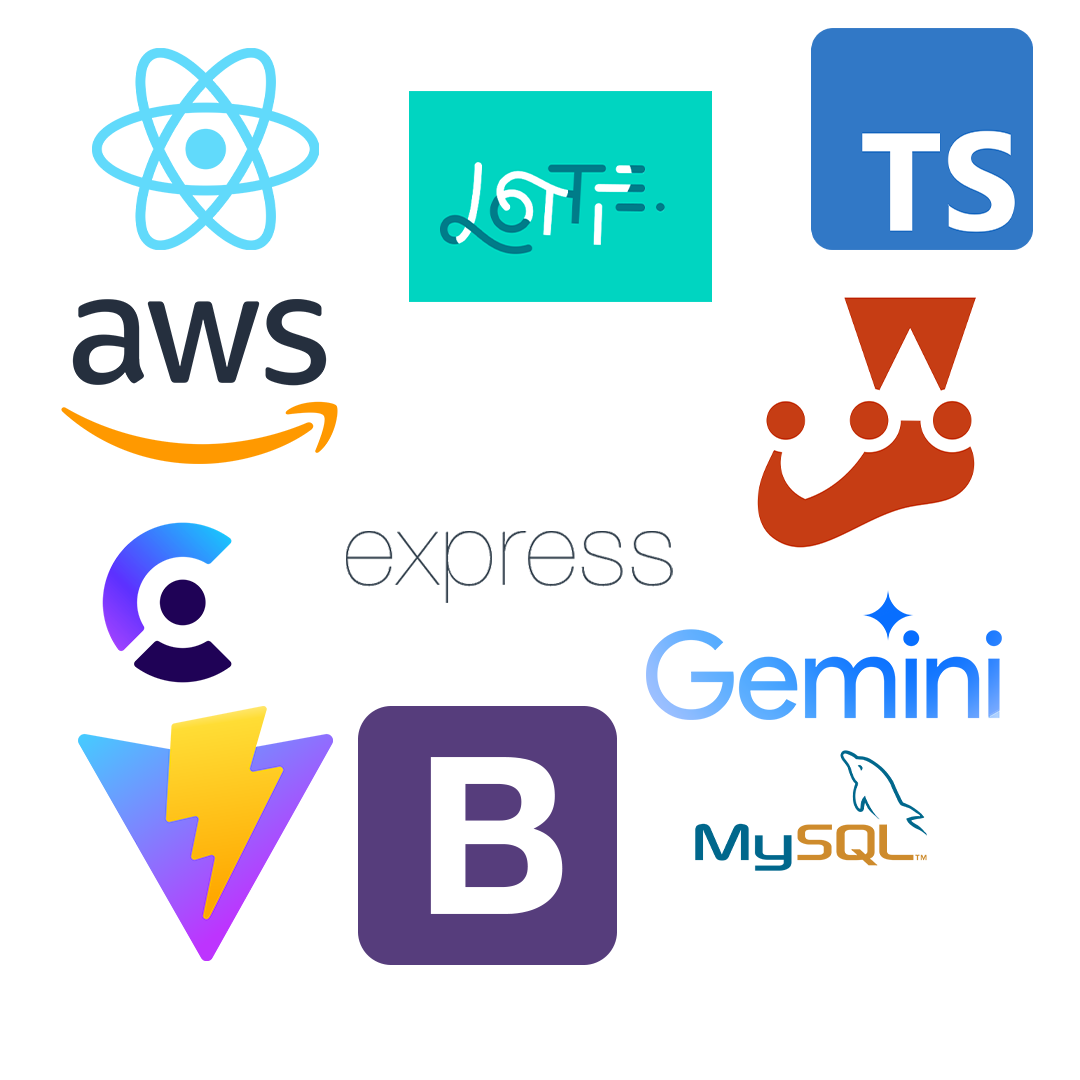
We used React with Vite TypeScript, JavaScript, CSS, Bootstrap, Lottie MySql Jest and SuperTest Express Gemini AI Clerk for authentication AWS for deployment and hosting We chose this stack for its reliability and scalability. Vite and React enable fast development and smooth user experiences. TypeScript adds type safety, catching errors early and improving code quality. Bootstrap helps create a responsive and consistent design, while Lottie adds smooth, interactive animations. MySQL offers a robust solution for data storage, and Jest with SuperTest ensures comprehensive testing for a stable application. Express provides a lightweight and efficient backend. Gemini AI enables us to generate quiz questions from various documents, making the process dynamic and smart. Clerk simplifies authentication, ensuring secure user management. AWS handles deployment and hosting, providing reliability and scalability. Overall, we wanted to challenge ourselves by diving into new, widely-used technologies to expand our skillset.
Challenges Faced
Learning a new language and new technologies in a short amount of time. AWS instances didn’t always work as expected. For example, the server occasionally needed to be rebooted, and we had to be mindful of the limitations of the free tier, which could lead to performance issues or additional costs.
Student Projects – Study BuddAI
A project made by Team Study BuddAI – An on the go learner App
Student Projects – Text to Speak
A project created by Team Slick’s Splendiferous Solo Services – Speak, without speaking.
Student Projects – Handy
A project made by Team C3PO – Find local, Fix Fast
Student Projects – Budgee
A project created by Team Budgee – See it. Track it. Own it.
Student Project – 2Gather
A project made by Team 2Gather – Friends Forever
Student Projects – The Travel Diary
A project created by The spice boys – Your one stop travel itinerary app, see where life takes you!
Student Projects – Knowva
A project made by Team Knowva – Gamify Your Learning, Personalise Your Progress
Student Projects – Wordslinger
A project created by Team Cowboys and Coders – Have you ever wated to learn a language or shoot a gun? Well hell, why choose?
Student Projects – Trekking Buddies
A project created by 404-team-name-not-found – Finding the perfect trekking partner just got easier!
Student Projects – Safespace Parking
A project created by Team Fab – Parking made easier, safer, and smarter.
Student Projects – Rescue Me
A project made by Team JJust HAK – Rescue them, Heal them, Take care of them!
Student Projects – Plant Zone
A project made by Team Plant People – Turning anyone into a plant person.